There is an urgent need for a mechanism for precisely identifying the battery’s state of charge (SOC), as LiFePO4 (LFB) batteries are being used in more portable devices. In this paper, the SOC in discharging state is estimated using an RBF neural network, an orthogonal least squares (OLS) algorithm, and an adaptive genetic algorithm (AGA). The OLS method is used to estimate the number of nodes in the hidden layer of the RBF neural network. The RBF’s parameters, such as RBF centers and RBF width, are changed using the AGA once the optimal neural network structure has been identified. The battery’s capacity is then predicted using an RBF neural network that has been trained on the SOC of an LFP battery. LFP batteries are used to test the applicability of the proposed estimation method in different discharge scenarios. In terms of efficiency, the proposed approach is compared with the backpropagation neural network (BP) and Coulomb integration method. According to the results, the proposed strategy outperforms the other methods already available.
Using a hybrid, estimate the state of charge for an LFP battery
written by Engineer's Planet
581
1 comment
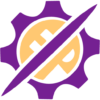
Engineer's Planet
We are establishing a streamlined platform tailored to the distinct requirements of engineering students and researchers across all disciplines. With a team of industry experts in every technological domain, we’re committed to turning your concepts into executable achievements.
1 comment
[…] Abstract […]